Original session date: 5 May2022
Topics covered: Graph Builder, Model Screening, Neural Networks
Speaker: Peter Polito (@peter_polito), Sr. Systems Engineer
Data: IODP Expd. 308—https://mlp.ldeo.columbia.edu/data/timeline/html/308.html GC955 —https://brg.ldeo.columbia.edu/data/ghp/JIP2/GC955-H/index.html?
The concept of pore pressure is a bit like the phases of the moon—most folks know there are phases, they're about a month long, there is a new moon, full moon, and stuff in between, but if you ask someone to explain why there are phases, most people get a bit stuck. Pore pressure is widely known about and most geoscientists can speak knowledgably about it but if you begin to ask more complex questions, we get a bit flummoxed. Pore pressure is very important to understand, one could argue that of all the data types collected by E&P organizations, pore pressure is the one bit of data that should be widely shared. They more we know, the more we can understand, the better our predictive models will be, and the safer drilling operations will be.
In this episode of JMP in the Upstream we dive into the concept of pore pressure, why it is important in drilling, how to easily visualize, and finally, how to predict it. Speaker Peter Polito uses data from IODP Expedition 308 and the GOM^2 drilling operation at GC955 to walk through these methods. He pulls porosity, gamma, density, and resistivity logs from three wells (two in the Ursa Basin and one in the Brazos-Trinity region) to build three unique predictive models using XGBoost, Boosted Tree, and Boosted Neural Networks (BNN). Although the R^2 value is lower for the Boosted NN, once compared to the actual pore pressure, the BNN provides a more stable prediction. When applied to the four remaining wells (one in the Ursa Basin, two in the Brazos-Trinity region, and one at GC955) the results are mixed. For wells that have a fairly stable pore pressure increase, the models performs very well. However, a fraction of the wells had high pressure kicks at depth, which neither model could handle and GC955 is such a different geologic setting, that the model does very poorly—the well is deeper, encounters different geology, and has an abundance of methane hydrate. While a great exercise in model building, Peter shows that in order to improve model quality, additional data is needed.
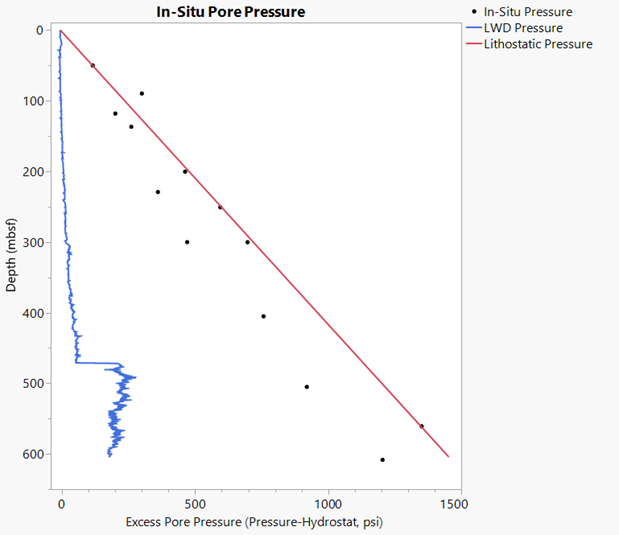
Figure 1. Excess pore pressure at U1324A, where logging tools encounter a large pore pressure kick around 480 m (blue line) but in-situ pore pressure measurements (black dots) suggest then entire depth is over pressured.

Figure 2. Comparison of the three predictive models compared to measured pressure at U1324A. XGBoost (blue) is the noisiest and Boosted Neural Network (green) while having the lowest R2, is devoid of large inaccurate pressure spikes, with Boosted Tree (red) falling in the middle.